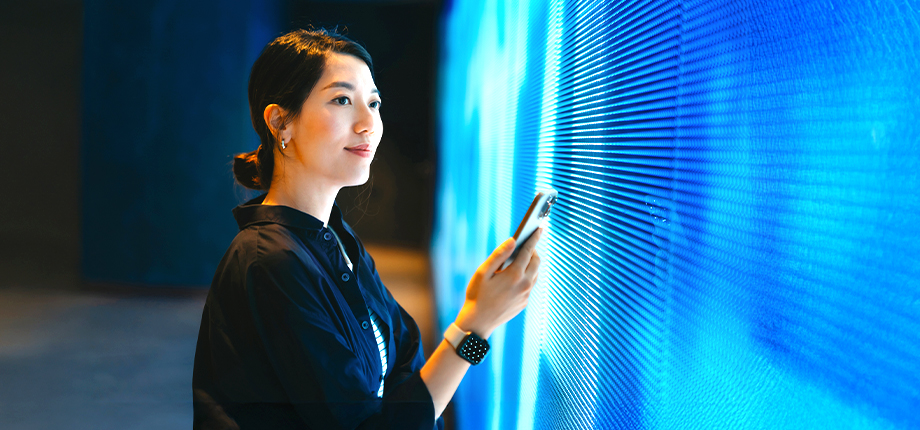
Dormant fraud, sleeper fraud, trojan horse fraud . . . whatever you call it, it’s an especially insidious form of account takeover fraud (ATO) that fraud teams often can’t detect until it’s too late. Fraudsters create accounts with stolen credentials or gain access to existing ones, onboard under the fake identity, then lie low, waiting for an opportunity to attack.
It takes a strategic approach to defeat the enemy from within, and fraudsters assume you won’t have the tools in place to even know where to start.
Dormant fraud uncovered: A case study
NeuroID, a part of Experian, has seen the dangers of dormant fraud play out in real time.
As a new customer to NeuroID, this payment processor wanted to backtest their user base for potential signs of fraud. Upon analyzing their customer base’s onboarding behavioral data, we discovered more than 100K accounts were likely to be dormant fraud. The payment processor hadn’t considered these accounts suspicious and didn’t see any risk in letting them remain active, despite the fact that none of them had completed a transaction since onboarding.
Why did we flag these as risky?
- Low familiarity: Our testing revealed behavioral red flags, such as copying and pasting into fields or constant tab switching. These are high indicators that the applicant is applying with personally identifiable information (PII) that isn’t their own.
- Fraud clusters: Many of these accounts used the same web browser, device, and IP address during sign-up, suggesting that one fraudster was signing up for multiple accounts. We found hundreds of clusters like these, many with 50 or more accounts belonging to the same device and IP address within our customer’s user base.
It was clear that this payment processor’s fraud stack had gaps that left them vulnerable. These dormant accounts could have caused significant damage once mobilized: receiving or transferring stolen funds, misrepresenting their financial position, or building toward a bust-out.
Dormant fraud thrives in the shadows beyond onboarding. These fraudsters keep accounts “dormant” until they’re long past onboarding detection measures. And once they’re in, they can often easily transition to a higher-risk account — after all, they’ve already confirmed they’re trustworthy. This type of attack can involve fraudulent accounts remaining inactive for months, allowing them to bypass standard fraud detection methods that focus on immediate indicators.
Dormant fraud gets even more dangerous when a hijacked account has built trust just by existing. For example, some banks provide a higher credit line just for current customers, no matter their activities to date. The more accounts an identity has in good standing, the greater the chance that they’ll be mistaken for a good customer and given even more opportunities to commit higher-level fraud.
This is why we often talk to our customers about the idea of progressive onboarding as a way to overcome both dormant fraud risks and the onboarding friction caused by asking for too much information, too soon.
Progressive onboarding, dormant fraud, and the friction balance
Progressive onboarding shifts from the one-size-fits-all model by gathering only truly essential information initially and asking for more as customers engage more. This is a direct counterbalance to the approach that sometimes turns customers off by asking for too much too soon, and adding too much friction at initial onboarding. It also helps ensure ongoing checks that fight dormant fraud. We’ve seen this approach (already growing popular in payment processing) be especially useful in every type of financial business. Here’s how it works:
- A prospect visits your site to explore options. They may just want to understand fees and get a feel for your offerings. At this stage, you might ask for minimal information — just a name and email — without requiring a full fraud check or credit score. It’s a low commitment ask that keeps things simple for casual prospects who are just browsing, while also keeping your costs low so you don’t spend a full fraud check on an uncommitted visitor.
- As the prospect becomes a true customer and begins making small transactions, say a $50 transfer, you request additional details like their date of birth, physical address, or phone number. This minor step-up in information allows for a basic behavioral analytics fraud check while maintaining a low barrier of time and PII-requested for a low-risk activity.
- With each new level of engagement and transaction value, the information requested increases accordingly. If the customer wants to transfer larger amounts, like $5,000, they’ll understand the need to provide more details — it aligns with the idea of a privacy trade-off, where the customer’s willingness to share information grows as their trust and need for services increase. Meanwhile, your business allocates resources to those who are fully engaged, rather than to one-time visitors or casual sign-ups, and keeps an eye on dormant fraudsters who might have expected no barrier to additional transactions.
Progressive onboarding is not just an effective approach for dormant fraud and onboarding friction, but also in fighting fraudsters who sneak in through unseen gaps. In another case, we worked with a consumer finance platform to help identify gaps in their fraud stack. In one attack, fraudsters probed until they found the product with the easiest barrier of entry: once inside they went on to immediately commit a full-force bot attack on higher value returns. The attack wasn’t based on dormancy, but on complacency. The fraudsters assumed this consumer finance platform wouldn’t realize that a low controls onboarding for one solution could lead to ease of access to much more. And they were right.
After closing that vulnerability, we helped this customer work to create progressive onboarding that includes behavior-based fraud controls for every single user, including those already with accounts, who had built that assumed trust, and for low-risk entry-points. This weeded out any dormant fraudsters already onboarded who were trying to take advantage of that trust, as they had to go through behavioral analytics and other new controls based on the risk-level of the product.
Behavioral analytics gives you confidence that every customer is trustworthy, from the moment they enter the front door to even after they’ve kicked off their shoes to stay a while.
Behavioral analytics shines a light on shadowy corners
Behavioral analytics are proven beyond just onboarding — within any part of a user interaction, our signals detect low familiarity, high-risk behavior and likely fraud clusters. In our experience, building a progressive onboarding approach with just these two signal points alone would provide significant results — and would help stop sophisticated fraudsters from perpetrating dormant fraud, including large-scale bust outs.
Want to find out how progressive onboarding might work for you? Contact us for a free demo and deep dive into how behavioral analytics can help throughout your user journey.